Neural Networks: Revolutionizing the Tech Industry
22 September 2024 by Sophie W.Neural networks have been at the forefront of revolutionizing the tech industry in recent years. With their ability to mimic the human brain and process complex data sets, they have become an essential tool in various fields such as healthcare, finance, and marketing. This article explores the key components of neural networks, their applications in different industries, as well as the challenges and limitations they face. Additionally, it delves into the future prospects and innovations that are driving this technology forward.
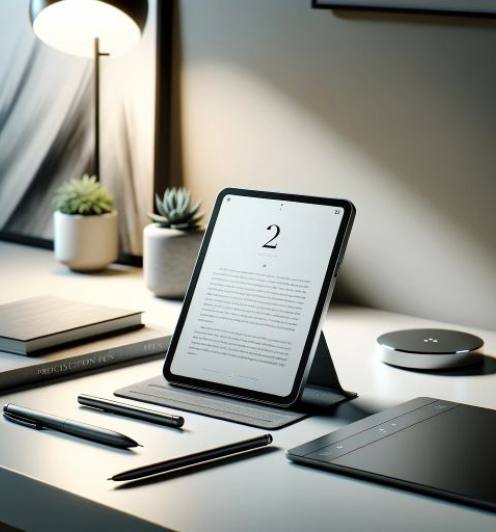
Neurons: Neurons are the basic building blocks of a neural network. They receive input, process it, and send output to other neurons.
Weights: Weights are assigned to the connections between neurons and determine the strength of the connection. These weights are adjusted during the training process to optimize the network's performance.
Activation function: The activation function determines whether a neuron should be activated or not based on the input it receives. Common activation functions include sigmoid, tanh, and ReLU.
Layers: Neural networks are typically organized into layers, with each layer performing a different type of processing. The three main types of layers are input layers, hidden layers, and output layers.
Bias: Bias is an additional input to each neuron that allows the network to learn complex patterns that may not be apparent in the input data alone.
Loss function: The loss function measures how well the neural network is performing by comparing its output to the true labels in the training data. The goal is to minimize the loss function during the training process. Understanding these key components is essential for building and training effective neural networks that can be applied to a variety of tasks in industries such as healthcare, finance, and technology.
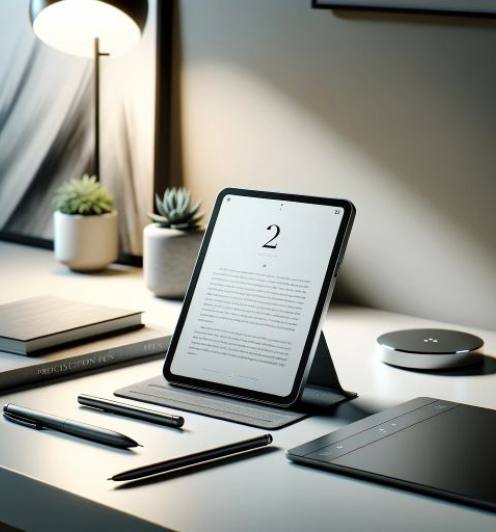
Introduction to Neural Networks
Neural networks, also known as artificial neural networks, are revolutionizing the tech industry with their ability to mimic the human brain's decision-making process. These complex algorithms have the capacity to learn from data, recognize patterns, and make predictions or decisions based on that information. This capability is what sets neural networks apart from traditional computer programs, making them powerful tools for a wide range of applications across various industries. In this article, we will delve deeper into the key components of neural networks, explore their applications in different sectors, discuss the challenges and limitations they face, and look at the future prospects and innovations in this exciting field.Key Components of Neural Networks
There are several key components that make up a neural network, including:Neurons: Neurons are the basic building blocks of a neural network. They receive input, process it, and send output to other neurons.
Weights: Weights are assigned to the connections between neurons and determine the strength of the connection. These weights are adjusted during the training process to optimize the network's performance.
Activation function: The activation function determines whether a neuron should be activated or not based on the input it receives. Common activation functions include sigmoid, tanh, and ReLU.
Layers: Neural networks are typically organized into layers, with each layer performing a different type of processing. The three main types of layers are input layers, hidden layers, and output layers.
Bias: Bias is an additional input to each neuron that allows the network to learn complex patterns that may not be apparent in the input data alone.
Loss function: The loss function measures how well the neural network is performing by comparing its output to the true labels in the training data. The goal is to minimize the loss function during the training process. Understanding these key components is essential for building and training effective neural networks that can be applied to a variety of tasks in industries such as healthcare, finance, and technology.